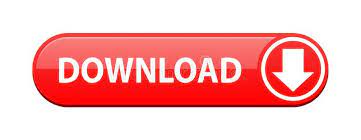


Incidentally, if you haven’t read Bill’s post go and read it it is one of the best explanations I have ever seen for a split-plot analysis.
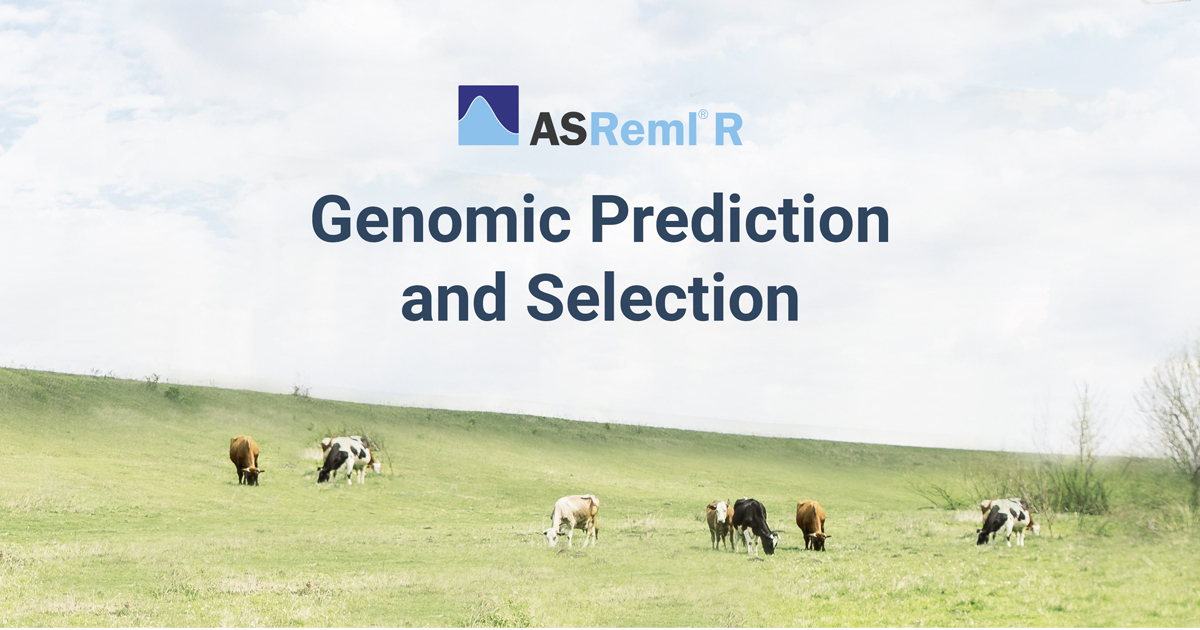
In addition, I will follow Bill Venables’s excellent advice and create additional variables for main plot and subplots, as it is confusing to use the same factor for two purposes (e.g. We can get the dataset from the MASS package, after which it is a good idea to rename the variables using meaningful names. I will use a traditional dataset to show examples of the notation for the three packages: Yates’ variety and nitrogen split-plot experiment. Besides researchers, the main users of ASReml/asreml-r are breeding companies.Īll these three packages are available in Windows, Linux and OS X. The last drawback is relative it is possible to freely use asreml for academic purposes (and there is also a version for developing countries). Main drawbacks are i- limited functionality for non-Gaussian responses, ii- it does not cover non-linear models and iii- it is non-free (as in beer an speech).
#PREDICT ASREML PLUS#
On the plus side it i- deals well with cross-classified random effects, ii- copes very well with pedigrees, iii- can work with fairly large datasets, iv-can run multivariate analyses and v- covers a large number of covariance and correlation structures. It is possible to fit pedigrees using the mmpedigree package, but I find the combination a bit flimsy.ĪSReml-R is, unsurprisingly, an R package interface to ASReml. Drawbacks: similar to nlme’s, but dropping point i- and adding that it doesn’t deal with covariance and correlation structures yet. On the positive side, it seems to be a bit faster than nlme and it deals a lot better with cross-classified random factors.
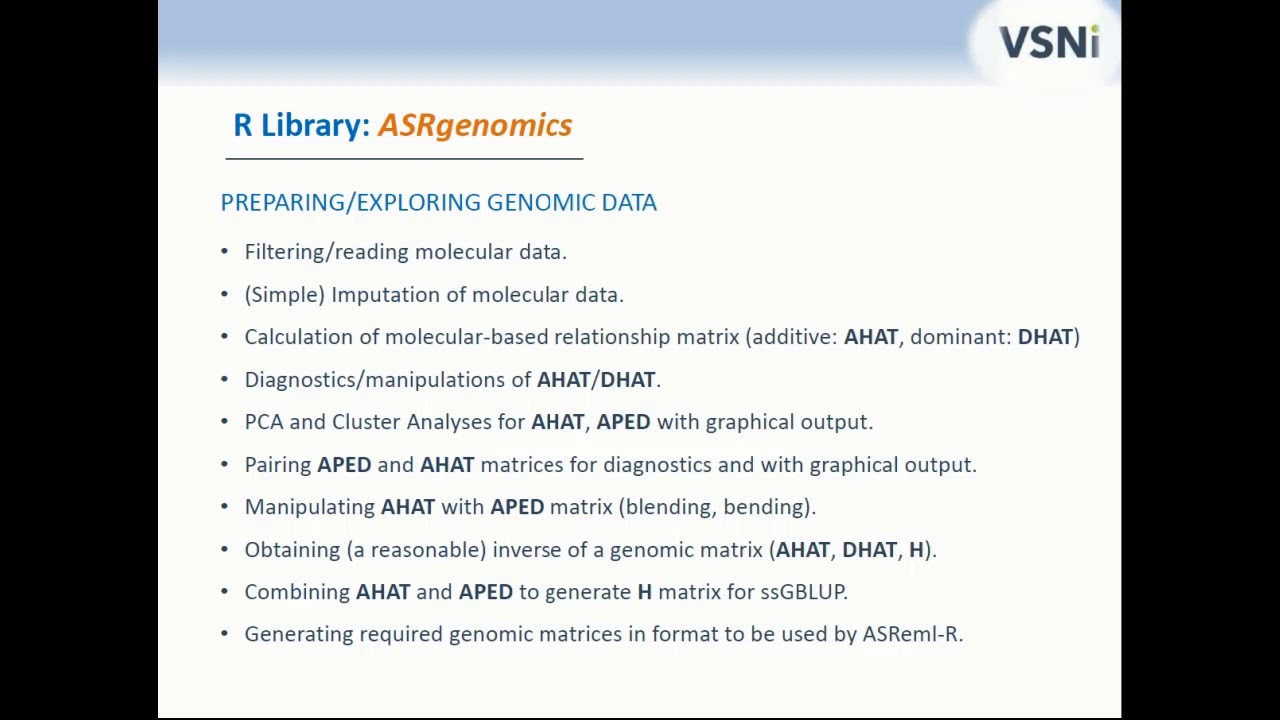
#PREDICT ASREML CODE#
Lme4 is a project led by Douglas Bates (one of the co-authors of nlme), looking at modernizing the code and making room for trying new ideas. In my opinion, its main drawbacks are i- fitting cross-classified random factors is a pain, ii- it can be slow and may struggle with lots of data, iii- it does not deal with pedigrees by default and iv- it does not deal with multivariate data. Its main advantages are, in my humble opinion, the ability to fit fairly complex hierarchical models using linear or non-linear approaches, a good variety of variance and correlation structures, and access to several distributions and link functions for generalized models. In addition to fitting hierarchical generalized linear mixed models it also allows fitting non-linear ones. Nlme is the most mature one and comes by default with any R installation. There are also several options for Bayesian approaches, but that will be another post. I will only mention nlme (Non-Linear Mixed Effects), lme4 (Linear Mixed Effects) and asreml (average spatial reml). OptionsĪs for many other problems, there are several packages in R that let you deal with linear mixed models from a frequentist (REML) point of view. At the end of 2005 I started using OS X and quickly realized that using a virtual machine or dual booting was not really worth it, so I dropped SAS and totally relied on R in 2009. Around 1999, I started playing with R (prompted by a suggestion from Rod Ball), but I didn’t really use R/S+ often enough until 2003. I was still using SAS for data preparation, but all my analyses went through ASReml (for which I wrote the cookbook), which was orders of magnitude faster than SAS (and could deal with much bigger problems). At the end of 1996 (or was it the beginning of 1997) I started playing with ASReml (programed by Arthur Gilmour mostly based on theoretical work by Robin Thompson and Brian Cullis). At that time (1995-1996) I moved to DFREML (by Karen Meyer, now replaced by WOMBAT) and AIREML (by Dave Johnson, now defunct-I mean the program), which were designed for the analysis of animal breeding progeny trials, so it was a hassle to deal with experimental design features. A brief history of timeĪt the beginning (1992-1995) I would use SAS (first proc glm, later proc mixed), but things started getting painfully slow and limiting if one wanted to move into animal model BLUP. The bulk of my use of mixed models relates to the analysis of experiments that have a genetic structure. A substantial part of my job has little to do with statistics nevertheless, a large proportion of the statistical side of things relates to applications of linear mixed models.
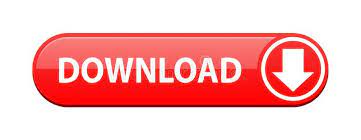